Publication: Deep Learning Insights on RV Function from ECG
In a groundbreaking study published on 29 December 2023 in the Journal of the American Heart Association, AIMS researchers have unveiled a novel approach to assess right ventricular (RV) size and function using deep learning-enabled electrocardiogram (ECG) analysis. Traditionally, assessing metrics like right ventricular ejection fraction (RVEF) and end-diastolic volume (RVEDV) required advanced imaging techniques, but this study suggests a paradigm shift in cardiac assessment.
Led by Son Q Duong and his team, the study utilized a deep learning-ECG model trained to predict RV dilation and dysfunction, as well as numerical values of RVEDV and RVEF. The model was trained using data from the UK Biobank and fine-tuned within a multicenter health system, demonstrating robustness across different patient populations.
The results were striking. The deep learning model exhibited high accuracy in predicting RV dysfunction and dilation, with area under the receiver operating characteristic curve values ranging from 0.77 to 0.91 across different cohorts. Moreover, the predicted RVEF showed a significant association with transplant-free survival, indicating its potential clinical relevance in prognosticating outcomes for patients with cardiovascular conditions.
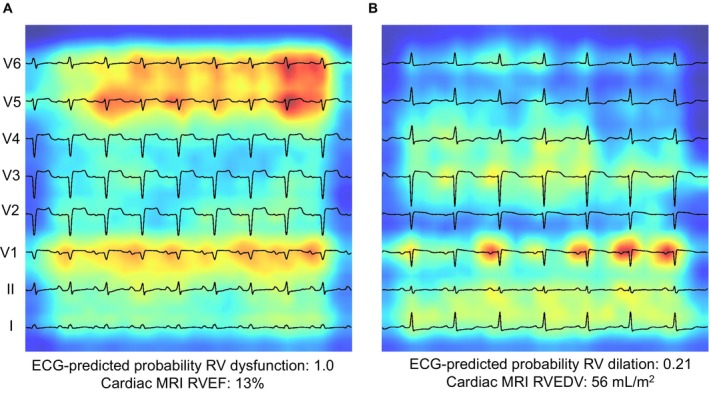
Saliency mapping ECG examples from the MSHoriginal test set. Leads are arranged from bottom to top: lead I, II, V1‐V6. Increasing shades of red indicate increasingly influential pixels in the model. A shows a true‐positive example of RV dysfunction. The P and QRS portions of V1, V5, and V6 are particularly influential. B shows a true‐negative example of RV dilation. QRS complex of lead I and P‐wave in V1 is particularly influential. MRI indicates magnetic resonance imaging; RVEF, right ventricular ejection fraction; and RVEDV, right ventricular end‐diastolic volume.